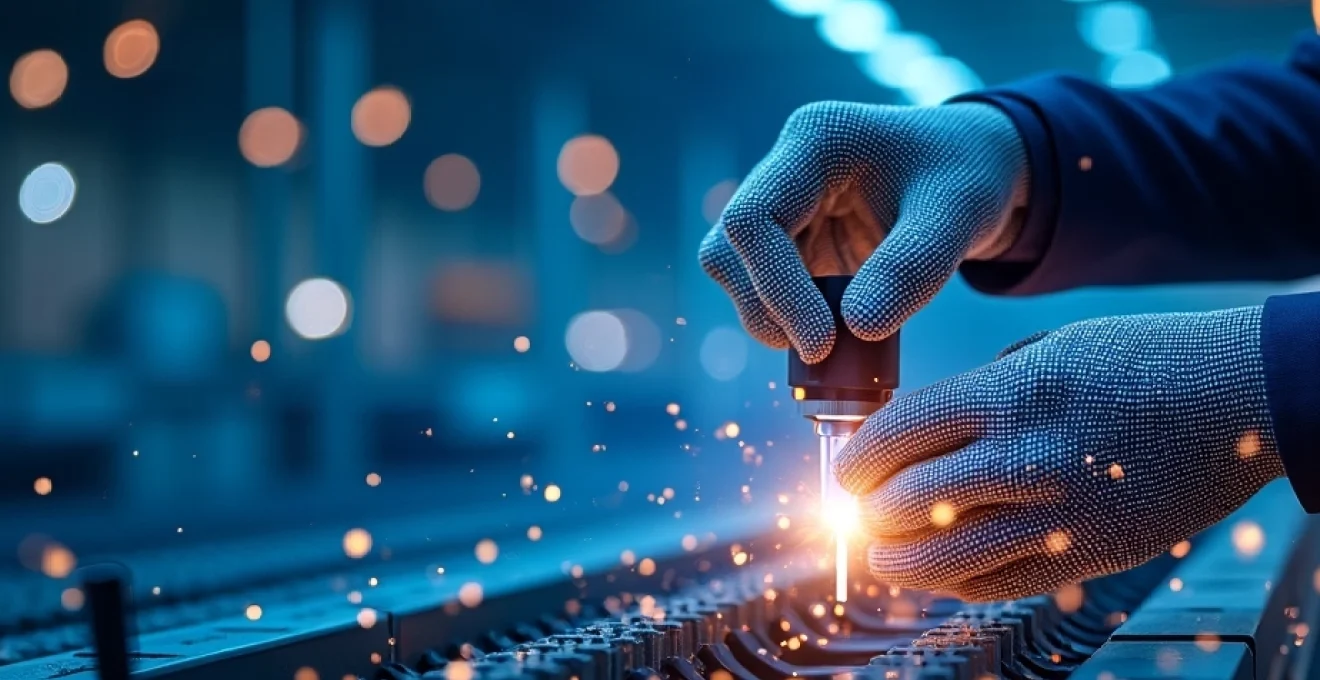
The manufacturing landscape is undergoing a radical transformation. As we progress deeper into the era of Industry 4.0, intelligent production systems are emerging as the cornerstone of modern manufacturing. These advanced systems leverage cutting-edge technologies to create smarter, more efficient, and highly responsive production environments. By integrating artificial intelligence, the Internet of Things, and advanced robotics, manufacturers are unlocking new levels of productivity, quality, and innovation. This shift towards intelligent production is not just an incremental improvement—it's a revolutionary change that's reshaping the very foundations of how we conceive, design, and produce goods.
Industry 4.0 and the evolution of intelligent manufacturing
Industry 4.0, often referred to as the fourth industrial revolution, marks a pivotal shift in manufacturing paradigms. This new era is characterized by the fusion of digital and physical systems, creating what we now call "smart factories." At the heart of this transformation lies the concept of intelligent production systems—a network of interconnected machines, devices, and software that work in harmony to optimize every aspect of the manufacturing process.
The evolution towards intelligent manufacturing didn't happen overnight. It's the result of decades of technological advancements, from the introduction of programmable logic controllers (PLCs) in the 1960s to the rise of computer-integrated manufacturing in the 1980s. However, the real game-changer has been the exponential growth in computing power and connectivity over the past decade.
Today's intelligent production systems are capable of self-optimization, self-configuration, and even self-diagnosis. They can adapt to changing conditions in real-time, predict maintenance needs before breakdowns occur, and seamlessly integrate with supply chain systems for just-in-time production. This level of autonomy and intelligence is revolutionizing manufacturing efficiency and agility.
But what exactly makes these systems "intelligent"? It's the combination of advanced sensors, sophisticated algorithms, and powerful data analytics that enables machines to perceive their environment, learn from experience, and make decisions. This mimics human cognitive functions but at a scale and speed that far surpasses human capabilities.
Core components of intelligent production systems
Intelligent production systems are built on a foundation of several key technologies, each playing a crucial role in creating a smart, interconnected manufacturing environment. Let's delve into these core components and understand how they contribute to the overall intelligence of modern production systems.
Industrial internet of things (IIoT) integration
The Industrial Internet of Things (IIoT) is perhaps the most fundamental component of intelligent production systems. IIoT refers to the network of interconnected sensors, instruments, and other devices networked together with industrial applications, including manufacturing and energy management. This connectivity allows for data collection, exchange, and analysis on a scale never before possible in manufacturing.
In an intelligent production system, IIoT enables machines, products, and even raw materials to communicate with each other and with central control systems. This constant flow of data provides real-time visibility into every aspect of the production process. For example, sensors on a production line can continuously monitor factors like temperature, pressure, and vibration, alerting operators to potential issues before they become problems.
The true power of IIoT lies in its ability to create a digital thread that connects every stage of the product lifecycle, from design and production to distribution and service. This comprehensive view allows manufacturers to optimize processes, reduce downtime, and improve product quality in ways that were previously impossible.
Machine learning and AI-driven process optimization
Artificial Intelligence (AI) and Machine Learning (ML) are the brains behind intelligent production systems. These technologies enable manufacturing systems to learn from data, identify patterns, and make decisions with minimal human intervention. In the context of manufacturing, AI and ML are used for a wide range of applications, from predictive maintenance to quality control and supply chain optimization.
One of the most impactful applications of AI in manufacturing is in process optimization. AI algorithms can analyze vast amounts of production data to identify inefficiencies and suggest improvements. For instance, an AI system might analyze the performance of different machine settings across thousands of production runs to determine the optimal configuration for a particular product.
Machine learning models can also adapt to changing conditions in real-time. As new data comes in, these models can update their predictions and recommendations, ensuring that the production system is always operating at peak efficiency. This level of adaptability is crucial in today's fast-paced manufacturing environment, where product specifications and market demands can change rapidly.
Advanced robotics and cobots in manufacturing
Robotics has been a part of manufacturing for decades, but the latest generation of robots—including collaborative robots or "cobots"—are taking automation to new heights. These advanced robots are equipped with sophisticated sensors and AI capabilities, allowing them to work alongside humans safely and efficiently.
In intelligent production systems, robots are no longer limited to repetitive tasks on assembly lines. They can now perform complex operations, make decisions based on real-time data, and even learn new tasks through demonstration. For example, a cobot might use computer vision to inspect products for defects, adjusting its inspection criteria based on feedback from quality control data.
The integration of robotics with other smart factory technologies creates powerful synergies. Robots can communicate with IIoT sensors to adjust their operations based on environmental conditions. They can also interface with AI systems to optimize their movements and workflows continuously. This level of integration results in unprecedented levels of flexibility and efficiency in manufacturing processes.
Digital twin technology for virtual process modeling
Digital twin technology is a revolutionary concept that's gaining traction in intelligent manufacturing. A digital twin is a virtual representation of a physical object or system that uses real-time data to create simulations, predict performance, and optimize operations. In manufacturing, digital twins can be created for individual machines, entire production lines, or even whole factories.
The power of digital twins lies in their ability to provide a virtual sandbox for testing and optimization. Manufacturers can use digital twins to simulate different scenarios, test new processes, and predict outcomes without disrupting actual production. This capability is invaluable for troubleshooting, process improvement, and innovation.
For example, a manufacturer might use a digital twin of their production line to test the impact of a new machine or process change before implementing it in the real world. The digital twin can simulate months of production in a matter of hours, providing insights that would be impossible to gain through traditional methods.
Data analytics and real-time decision making in smart factories
At the heart of intelligent production systems is the ability to collect, analyze, and act on data in real-time. This capability transforms manufacturing from a reactive to a proactive process, where decisions are made based on predictive insights rather than historical data. Let's explore some key applications of data analytics in smart factories.
Predictive maintenance using sensor data
Predictive maintenance is one of the most impactful applications of data analytics in manufacturing. By analyzing data from IIoT sensors, AI algorithms can predict when a machine is likely to fail, allowing maintenance to be scheduled before a breakdown occurs. This approach can dramatically reduce downtime and maintenance costs.
For instance, vibration sensors on a piece of equipment can detect subtle changes that might indicate an impending failure. The AI system can analyze this data in conjunction with historical maintenance records, operating conditions, and even data from similar machines in other factories to predict when maintenance will be needed. This level of predictive capability can increase equipment uptime by 10-20%, resulting in significant cost savings and productivity improvements.
Quality control through computer vision and AI
Quality control is another area where intelligent production systems are making a significant impact. Advanced computer vision systems, powered by AI, can inspect products at speeds and levels of accuracy far beyond human capabilities. These systems can detect defects that might be invisible to the human eye, ensuring consistently high product quality.
AI-powered quality control systems can also adapt to new products or quality standards quickly. Machine learning algorithms can be trained on examples of defective and non-defective products, allowing them to recognize new types of defects as they emerge. This adaptability is crucial in industries where product specifications change frequently.
Supply chain optimization with blockchain integration
Intelligent production systems extend beyond the factory floor to encompass the entire supply chain. By integrating blockchain technology, manufacturers can create transparent, secure, and efficient supply chains. Blockchain provides an immutable record of every transaction and movement in the supply chain, from raw materials to finished products.
This level of transparency can help manufacturers optimize their supply chains in several ways. It can reduce fraud and errors, streamline customs processes, and enable real-time tracking of goods. For example, a manufacturer could use blockchain to verify the authenticity of raw materials, track the progress of shipments, and ensure compliance with regulatory requirements.
Moreover, blockchain integration can enable smart contracts—self-executing contracts with the terms directly written into code. These can automate many supply chain processes, such as payments and order fulfillment, further increasing efficiency and reducing costs.
Case studies: successful implementations of intelligent production systems
To truly understand the impact of intelligent production systems, it's valuable to look at real-world implementations. Many leading manufacturers across various industries have successfully adopted these technologies, reaping significant benefits in terms of efficiency, quality, and innovation.
One notable example is a major automotive manufacturer that implemented a comprehensive IIoT system across its production facilities. By connecting machines, tools, and products, the company was able to create a digital thread that provided real-time visibility into every aspect of production. This implementation resulted in a 15% increase in overall equipment effectiveness (OEE) and a 5% reduction in energy consumption.
Another case study involves a consumer electronics company that deployed AI-powered predictive maintenance systems. By analyzing data from thousands of sensors across their production lines, the company was able to predict equipment failures with over 90% accuracy. This predictive capability reduced unplanned downtime by 30% and maintenance costs by 25%.
In the aerospace industry, a leading manufacturer has leveraged digital twin technology to optimize its production processes. By creating virtual models of their entire production line, the company can simulate months of production in just a few hours. This capability has enabled them to identify and resolve potential issues before they occur in the real world, resulting in a 20% reduction in production cycle times and a 15% improvement in first-time-right production.
Challenges and considerations in adopting smart manufacturing technologies
While the benefits of intelligent production systems are clear, implementing these technologies is not without challenges. Organizations looking to adopt smart manufacturing technologies must navigate several key considerations to ensure successful implementation.
One of the primary challenges is the significant upfront investment required. Implementing IIoT systems, advanced robotics, and AI technologies can be costly, and it may take time to see a return on this investment. Organizations need to carefully evaluate the potential benefits against the costs and develop a clear implementation strategy.
Data security and privacy are also major concerns. With the increased connectivity of intelligent production systems comes increased vulnerability to cyber attacks. Manufacturers must implement robust cybersecurity measures to protect sensitive data and prevent disruptions to their operations.
Another consideration is the need for skilled workers who can operate and maintain these advanced systems. The shift towards intelligent manufacturing is creating demand for new skill sets, such as data science and AI engineering. Organizations need to invest in training their existing workforce and recruit new talent to bridge this skills gap.
Interoperability between different systems and technologies can also be a challenge. Many manufacturers have legacy systems that may not easily integrate with newer technologies. Ensuring seamless communication between different components of the intelligent production system is crucial for realizing its full benefits.
Future trends: edge computing and 5G in intelligent production
As intelligent production systems continue to evolve, emerging technologies are set to further enhance their capabilities. Two technologies that are poised to have a significant impact are edge computing and 5G networks.
Edge computing brings data processing closer to the source of data generation—in this case, the machines and devices on the factory floor. This approach reduces latency and enables real-time decision making, which is crucial in manufacturing environments where split-second decisions can have significant impacts. Edge computing also reduces the amount of data that needs to be sent to the cloud, improving efficiency and reducing bandwidth requirements.
The rollout of 5G networks is set to revolutionize connectivity in manufacturing environments. With its high speed and low latency, 5G will enable more devices to be connected simultaneously, facilitating the implementation of large-scale IIoT systems. It will also enable new applications that require ultra-reliable and low-latency communication, such as remote control of robotic systems.
Looking ahead, we can expect to see increased integration of these technologies in intelligent production systems. For example, 5G-enabled edge devices could process vast amounts of sensor data in real-time, enabling even faster and more accurate decision-making. This could lead to further improvements in efficiency, quality, and flexibility in manufacturing processes.
As these technologies mature and become more widely adopted, the vision of truly autonomous factories—where production systems can self-optimize and adapt to changing conditions without human intervention—is becoming increasingly achievable. This represents the next frontier in intelligent manufacturing, promising to deliver unprecedented levels of efficiency and innovation in the production of goods.